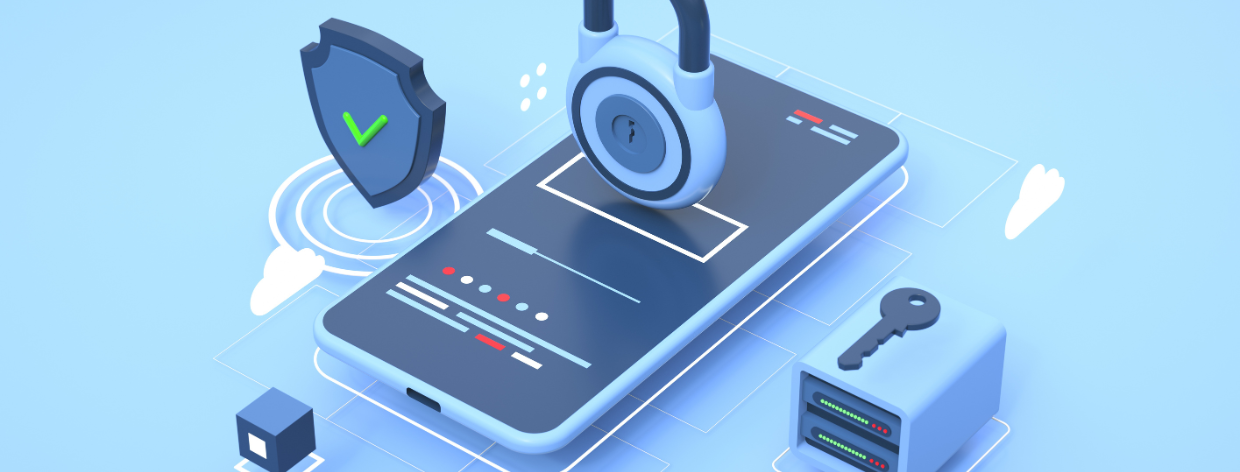
- 17th Feb 2024
- 11:54 am
A machine capable of interpreting, evaluating, analysing and making decisions like human beings is referred to as Artificial Intelligence (Ghillani, 2022). However, Artificial Intelligence (AI) surpasses the capabilities of the human brain by accomplishing tasks in large volumes in minimum time. The technology of Artificial Intelligence is overtaking many functions of businesses, and its importance lies in its accuracy and swiftness of carrying out tasks. Artificial Intelligence consists of the collection of data of different natures, which it then utilizes for performing its functions. As a result, various problems are registered related to data privacy and model security in AI research.
- Some of the common problems related to data privacy and model security of AI can be stated in the form of the vulnerability of attacks, evasion and class imbalance. Despite the minor nature of some of these problems, these have a dangerous impact on the data of individuals.
- The key motivation behind this paper was to understand and discuss the significance of findings in the form of challenges of data privacy and model security within AI.
- The key trends of literature in AI research can be enumerated by information security, predictive analytics development and models of language. Digital avatars and AI ethics are additional literature trends with regard to AI.
- The scope of the paper is to understand the reasons and challenges of data privacy and model security that make the implementation of such technologies vulnerable. The additional scope of the paper is to discuss the threats that result from the breach in data privacy and cyberattacks.
- Through this paper, I have made contributions by comparing different academic publications and analysing the shared characteristics between them as well as pointing out the difference of opinions in the same. Additionally, I have contributed by pointing out the strengths and weaknesses of the papers.
Main body
Overview of Data Privacy in AI
As per the views of Zhang & Lu (2021) in his research paper, there can be different definitions of Artificial Intelligence in various sectors. Many a time, AI is used as an interchangeable term in the place of Machine Learning and is, therefore, referred to as "big data" in many cases. AI for businesses can be defined as a set of technologies in the form of automated and analytics tools that are utilized by businessmen in order to perform several tasks of numerous natures.
The use of artificial intelligence and utilization of data go simultaneously. The utilization of data is essential for Artificial Intelligence to work in a smooth manner. As a result, data and artificial intelligence are intricate to each other (Fang, Qi & Wang, 2020). Data provides artificial intelligence information that such machines can utilize, and some knowledge in the form of data is personal and sensitive in nature.
Chen, Chen & Lin (2020) argued in his academic paper that the increased use of digitalization and the utilization of data in AI does not mean that such advanced technology makes use of sensitive information every time. This led to a key difference in the publication of this publication with that of the previous one. The increased use of data in AI has resulted in the growth of concerns on the part of people with this technology; because of this, the architects of AI are in a continuous process to minimize the collection of sensitive information. With the advancement of technology, the introduced technology of 5G has aimed to improve reliability and lowers the requirement of collecting sensitive data.
Xu, Xiang & He (2021), in his paper “Data Privacy Protection in News Crowdfunding in the Era of Artificial Intelligence”, stated that the broad design of AI is characterised by several areas which function by the collection of data of various natures. Input data is one such area that is fundamental for the working of every AI as it utilizes data in order to give it commands. Input data can be both personal as well as impersonal, and are important to use the technology of AI. At this stage, the use of production data is used in order to feed information to the AI system.
A research paper “Data Privacy Threat Modelling for Autonomous Systems” shared the characteristics of the previous paper and has stated that the input data is easiest to explain and the next area of the function of AI, which is represented by a stage called "black box", is challenging because of the invisibility of the functions being performed by AI (Azam, et al. 2021). In other words, the black-box stage can be defined as a processing stage in which the data provided in the input is processed by AI machines. This adds to the complexity of understanding the data privacy aspect of the technology.
Hao, et al.(2019) argued that the output data is the third area of the function of AI. Despite the knowledge of the input data and the understanding of the accurate processing of data, the outcome of the output can be surprising in many cases. This view has been supported in the academic paper “Data Privacy Threat Modelling for Autonomous Systems” shared similar thoughts about the data privacy of the previous paper and supported that the privacy of data in output mode can sometimes be unpredictable, which raises the probability of sensitive information being generated. Additionally, the use of hybrid data can prove to be dangerous with regard to the privacy of the data.
Overview of Model Security in AI
The primary function of model security in Artificial Intelligence, as has been described in the academic paper “Deep Learning and Artificial Intelligence Framework to Improve the Cyber Security”, is to safeguard the model functionality of AI by preventing attackers from harming them. Model security refers to a security system that is designed to protect valuable information from individuals who might cause harm (Ghillani, 2022). The AI model security systems are essential for every user of Artificial Intelligence because of their accurate analysis and interpretation of a situation and immediate response time. AI models are implemented and used with the aim of detecting variations and unfamiliar patterns that may be entitled to a security violation.
The statements given in the academic paper “How to Leverage AI for Security Enhancement?” are also of similar opinions in which it has been stated that the security threats faced by organisations are a continuous process and the strategies of cyber attackers keep on changing; however, the patterns of their attacks have some similarities (Fang, Qi & Wang, 2020). This can neither be understood nor interpreted by security officials. This is where the implementation of AI model security systems comes into play. Because of the detection of the simplest and the most meagre changes in the security system, AI model security reduces the duplicative process of cyber attacks.
In the opinions of Manheim & Kaplan (2019) in his research paper, Artificial Intelligence models are used to critically inspect a network setup within an organisation and discover abnormal patterns, such as sensing unfamiliar network scanning from an unfamiliar IP address that may cause harm to the sensitive information and cause an alert. Another way the AI models security is used is by detecting hijack of the username or password of a particular username. Research paper “AI-Driven Cybersecurity” has shared the characteristics of the previous paper and has stated that the implementation of model security in AI improves the management of the vulnerability of networks. As most of the cyber attacks happen because of the takeover of network systems, the accuracy of the model security systems in the detection of the abnormal entrance of outsiders makes it a useful tool in the hand of people.
Letaief, et al. (2021) argued and differentiated the previous statements by saying that the use of real-time data by the AI model security enables such security systems to review websites and other security news by using text analysis which makes it possible for the AI security models to present security breaches and threats in a more efficient manner. The self-learning potential of the AI has been discussed, which is a completely different statement from the other papers. According to this paper, the system of Artificial Intelligence model security is a smart technology which continues to learn by itself, which accelerates the development of security within the system.
Shared opinions of publications |
Differences of publications |
|
|
Challenges of Data privacy and Model Security in AI
In the academic paper “Data Privacy Threat Modelling for Autonomous Systems”, Azam, et al. (2021) opined that Artificial Intelligence is only a technology and it is vulnerable to cyber attacks every time. The strengths of the paper are in the explanation of the processes which have the capability to harm the security of AI. The use of Artificial Intelligence is the result of the accumulation of data that is mostly personal in nature, which increases the concern of many people and organisations to utilize this system. However, the large amount of data and information proves to be difficult for organisations to neglect the technology of AI. The main weakness identified in the paper is the insufficient examples provided by the authors with regard to the issues of data privacy.
Sarker, Furhad & Nowrozy (2021) in his research paper “AI-Driven Cybersecurity”, argued that the use of AI comes with several challenges to data privacy as the collection of personal data by the systems of AI risks the rights and freedom of the individual. One of the challenges related to data privacy in AI is the imbalance of class which can be defined as a binary confusion. Imbalance of class refers to the uneven favour of a specific class, the description of which is one of the strengths of this publication. At an initial level, this imbalance seems to be minor and does not seem dangerous, which is why the architects of AI seem to be ignorant of this fact. However, the favour of a specific class can prove to be inaccurate in producing results and lead to an issue from the perspective of data privacy. One of the weaknesses of this paper was in the insufficiency of details provided concerning the issue of class imbalance.
The digital system of AI comes with the challenge of the vulnerability of attacks which has the potential to confuse the system and threaten the data of an organisation (Chen, Chen & Lin (2020). As a result, this challenge is severe, which leads to growing concern among people about the utilization of this system. The utilization of relevant examples for proving the relationship between data privacy and attacks on AI systems is the strength of this academic paper. However, the paper only discussed the relevance of the attacks on AI and failed to provide any other additional information, which becomes the weakness of the paper.
Figure 1: Cyber Attacks on AI
(Source: Checkpoint, 2023)
As per the views of Alhayani, et al. (2021) in his paper, one of the challenges of the AI security model is to understand the cause of the triggering of an alarm that indicates a security breach. Having knowledge about the triggering of any security breach can enable organisations to face a threat in an effective manner. The strength of the paper is in the requirement of knowledge on the human level, which can equip individuals to monitor and correct any issues in the case of any threat. However, the lack of techniques and examples leaves the understanding of the topic incomplete, which becomes its weakness.
The strength of academic paper “Data Privacy Threat Modelling for Autonomous Systems” is in its description of the model security challenge of evasion, which is referred to as changing the input and confusing the AI model security. This is one of the most common attacks chosen by attackers (Azam, et al. 2021). However, insufficient numbers of surveys lack in the authentication of the information provided in the article, which results in the weakness.
As per the view of Sarker, Furhad & Nowrozy (2021) in research paper “AI-Driven Cybersecurity”, one of the critical challenges associated with data privacy and model security in AI research is the privacy consideration of the data. This challenge raises a number of questions in regard to information privacy in AI technology. The traditional notions of privacy have been challenged in the context of AI research. The key strength of this research paper is that it provides authentic information about data protection which helps people to protect their data. On the other side, the weakness of this research paper is that it doesn't include an effective survey process which has a significant impact on the outcomes.
At the same time, it has been criticized by Sarker, Furhad & Nowrozy (2021) that the biased and discriminated data is also considered as the main challenge that hampers the privacy of information. It can be said that the AI system is only good when the data is trained. On the other hand, if the data is biased, it can make the entire AI system biased, which can lead to the unfair treatment of individuals. However, in regard to the strength of this research paper, it can be said that it facilitates proper knowledge about the use of AI to reduce the biased and discrimination in data. An adequate and appropriate understanding of the use of AI and model security helps individuals to protect their personal information from any cyber attack.
It has been analyzed that data scarcity is another challenge that affects the use of AI and model security in AI research. Most countries use local data for developing applications due to the lack of accurate data and resources (Sarker, Furhad & Nowrozy, 2021). Data is a very significant aspect of AI, and labelled data is used by individuals to attain the desired outcomes.
Conclusion
From the paper above, it can be concluded that the technology of artificial intelligence is an important factor in enjoying dominance in the digital world. The interpretation of large volumes of data also makes the utilization of this technology an important aspect. However, the use of AI also results in security concerns in the form of data privacy and model security. As a result, the technology poses numerous challenges and makes it vulnerable to outer attacks. The paper has pointed out different challenges related to data and model security that come in the way of the technology of Artificial Intelligence. Vulnerability to attacks is one such challenge which makes AI security prone to outer attacks. The challenge of evasion is the result of the hijacking of a network by attackers, which confuses the system and increases its vulnerability of the system.
The comparison between different academic papers has attempted to understand the challenges and threats resulting from the implementation of AI and model security systems. Moreover, on the basis of comparison and analysis between different academic papers discussed above, it can be concluded that data privacy and AI model security are severe challenges that need to be addressed immediately.
Reference
Alhayani, B., Mohammed, H. J., Chaloob, I. Z., & Ahmed, J. S. (2021). Effectiveness of artificial intelligence techniques against cyber security risks apply of IT industry. Materials Today: Proceedings, 531.
Azam, N., Michala, L., Ansari, S., & Truong, N. B. (2022). Data Privacy Threat Modelling for Autonomous Systems: A Survey from the GDPR's Perspective. IEEE Transactions on Big Data.
Berente, N., Gu, B., Recker, J., & Santhanam, R. (2021). Managing artificial intelligence. MIS quarterly, 45(3).
Checkpoint, (2023) Cyber Attacks on AI https://blog.checkpoint.com/2022/01/10/check-point-research-cyber-attacks-increased-50-year-over-year/
Chen, L., Chen, P., & Lin, Z. (2020). Artificial intelligence in education: A review. Ieee Access, 8, 75264-75278.
Fang, H., Qi, A., & Wang, X. (2020). Fast authentication and progressive authorization in large-scale IoT: How to leverage ai for security enhancement. IEEE Network, 34(3), 24-29.
Ghillani, D. (2022). Deep Learning and Artificial Intelligence Framework to Improve the Cyber Security. Authorea Preprints.
Hao, M., Li, H., Luo, X., Xu, G., Yang, H., & Liu, S. (2019). Efficient and privacy-enhanced federated learning for industrial artificial intelligence. IEEE Transactions on Industrial Informatics, 16(10), 6532-6542.
Letaief, K. B., Shi, Y., Lu, J., & Lu, J. (2021). Edge artificial intelligence for 6G: Vision, enabling technologies, and applications. IEEE Journal on Selected Areas in Communications, 40(1), 5-36.
Manheim, K., & Kaplan, L. (2019). Artificial intelligence: Risks to privacy and democracy. Yale JL & Tech., 21, 106.
Sarker, I. H., Furhad, M. H., & Nowrozy, R. (2021). Ai-driven cybersecurity: an overview, security intelligence modeling and research directions. SN Computer Science, 2, 1-18.
Xu, Z., Xiang, D., & He, J. (2021). Data Privacy Protection in News Crowdfunding in the Era of Artificial Intelligence. Journal of Global Information Management (JGIM), 30(7), 1-17.
Zhang, C., & Lu, Y. (2021). Study on artificial intelligence: The state of the art and future prospects. Journal of Industrial Information Integration, 23, 100224.