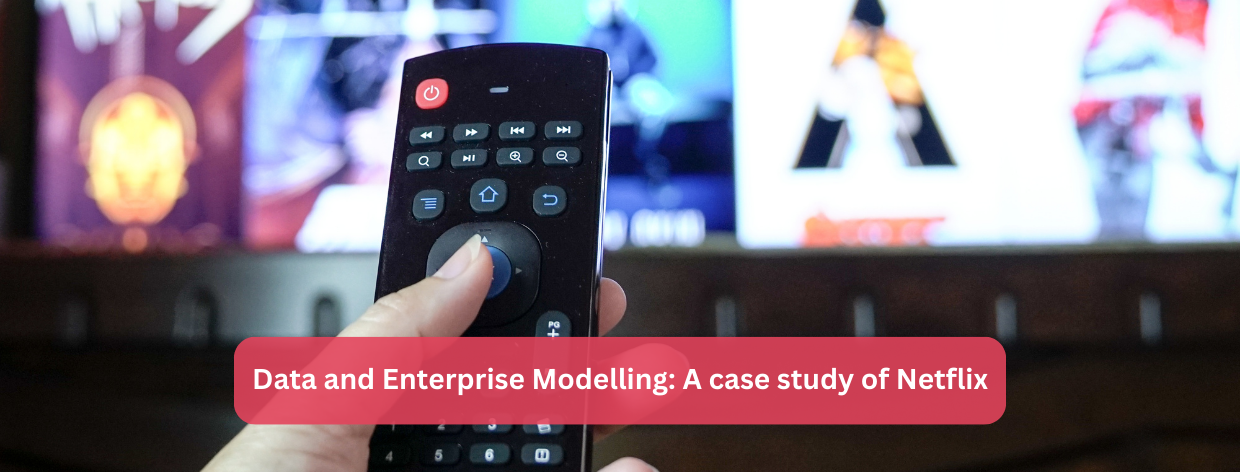
- 2nd Feb 2024
- 15:18 pm
- Admin
Abstract
Netflix's victory in the streaming sphere is anchored not just in content but firmly in its approach Data Resource Management (DRM). This research explored the intricacy of Netflix's DRM rules, from its pioneering recommendation algorithms to the difficulties of data-enterprise model integration. Through an analytical viewpoint, the study showed the vital role of DRM in gaining a competitive advantage, emphasising its relevance in user engagement, predictive analytics, and tailored content strategies. Concurrently, the findings spotlighted underlying issues, notably in scalability and integration, underscoring the challenge of handling enormous data in a dynamic global setting. The debate further questioned the balance Netflix maintains between micro-level data management and macro-level business strategy. Conclusively, while Netflix's DRM approaches serve as a blueprint for success in the streaming sector, they also indicate severe difficulties and areas for additional exploration in the field of data management.
Executive Summary - Data and Enterprise Modelling: A case study of Netflix
Netflix's superiority in the streaming sector is partly attributable to its superior Data Resource Management (DRM) strategies. This research illustrates the diverse effect of DRM in influencing Netflix's user experience and larger business perspective. Key findings underline the platform's algorithm-driven content recommendations, predictive analytics capabilities, and region-specific content personalization, all rooted in DRM. However, barriers exist in data-enterprise model integration, emphasising the intricacies of aligning massive data quantities with broader company strategy. Additionally, the paper underscores scalability concerns, underlining the restrictions of managing different data sources despite international progress. The financial advantage achieved by DRM, while currently robust, demands ongoing innovation, given the growing technology and competitive circumstances. The study culminates in a critical debate, advocating new research alternatives, especially in light of expanding technology and altering international norms.
1.0 Introduction
Overview of the Business Issues
The fast rise of streaming services has thrust data resource management (DRM) into the forefront for corporations like Netflix. One key DRM difficulty impacting Netflix is the convergence between Data Modelling and Enterprise Modelling. In a fiercely competitive sector, these frameworks are vital for Netflix to streamline its business operations, provide tailored user experiences, and remain flexible in its decision-making processes.
Figure: Netflix subscribers worldwide
Source: Statista, 2023
According to a report from Statista, as of October 11, 2023, Netflix has over 238.39 million paid subscriptions globally. This huge user base produces a gigantic number of data daily, making the administration, analysis, and use of this data a Herculean job. Poor DRM practices in the disciplines of Data and Enterprise Modelling could lead to inefficient use of resources, inferior customer service, and finally, a decline in market share. Given the company's huge scale and the complexities of data consumption, a comprehensive grasp of how Data Modelling interlinks with Enterprise Modelling is critical for Netflix to continue its industry leadership. This research attempts to go into the complexity and repercussions of these connected DRM problems inside the organizational context of Netflix.
Research Approach
Qualitative secondary research involves the analysis of existing data, publications, and resources to produce conclusions (Largan and Morris, 2019). This approach will be employed in this study to examine at Netflix's DRM difficulties in Data and Enterprise Modelling. The reason for performing qualitative secondary research is in its potential to deliver a nuanced knowledge of complex organizational difficulties without the time and budget limits normally associated with primary data collection. It permits for a complete examination of existing academic research, case studies, and organizational reports that give insights into the highlighted DRM difficulties. By merging already-available high-quality data, the research seeks to generate useful suggestions for Netflix.
2.0 Literature Review
2.1 Evolution of Data Resource Management (DRM) in Streaming Services
The progress of Data Resource Management (DRM) in the context of streaming services has been remarkable throughout the past decade. Initially, DRM in streaming was mostly concerned with digital rights and access control (Montoro?Pons et al. 2021). However, as the industry grew, the focus moved towards leveraging data for operational efficiency and customer experience development. A key essay by Abid (2022) investigated how streaming services started employing complex data algorithms for content selection, user segmentation, and pricing techniques.
According to a report by Deloitte, as of 2022, 80% of U.S. homes have at least one paid streaming subscription, emphasising the volume of data collected and the demand for efficient DRM (Deloitte, 2022). This expansion in user base has driven streaming companies to update and innovate their DRM methods periodically. The data is no longer merely an asset but a critical business engine that has to be maintained properly. Mololoth, Saguna and Åhlund (2023) demonstrated how big data analytics and machine learning have become key parts of DRM. These technologies not only aid in evaluating client behaviour but also help in predicting analytics, which further impacts business choices and content curation. The study found that Netflix, as an industry leader, spent substantially on DRM capabilities, especially in data modelling and analytics, to keep its competitive edge. The rise of DRM in streaming services has advanced beyond simple rights management to a sophisticated ecosystem involving data analytics, machine learning, and predictive modelling. These improvements in DRM techniques have been vital for organisations like Netflix to handle the volume of data they collect and remain ahead in a highly competitive sector.
2.2 Data Modelling Techniques in DRM
Data modelling in Data Resource Management (DRM) is an increasingly vital field of research, notably for its applications in promoting organizational efficiency. In his 2020 essay, Tshontsho covers numerous data modelling methodologies, such as Entity-Relationship (ER) models, Object-Oriented (OO) models, and Dimensional models. Each has its inherent benefits and disadvantages, dependent on the particular objectives and purposes of an organization. Significantly, a 2022 Gartner report reveals that 75% of businesses will shift from piloting to operationalizing AI by 2026, underscoring the demand for reliable data models in DRM to support these advanced technologies (Gartner, 2021). Integration of AI and machine learning algorithms into DRM depends on well-ordered data models, a characteristic which has been addressed at length by Bertoni et al. (2021). According to Lavin et al. (2022), well-defined data models enable quicker data intake and interpretation, hence becoming a need for successfully deploying machine learning algorithms for business intelligence.
The study of Bibri (2022) adds another layer to this issue by stressing the requirement of adaptive data modelling approaches. In the era of Big Data, static data models can be constrictive; thus, Brown advocates for flexible models that can expand with changing business demands and data environments. For a corporation like Netflix, the importance of data modelling methodologies in DRM becomes particularly clear. Netflix leans substantially on data analytics for content recommendation, audience segmentation, and even quality control. Given the company’s massive data quantities, implementing an efficient and adaptive data modelling method is not only beneficial but crucial. Implementing the relevant data models supports Netflix's DRM strategy, optimising both the utilisation of resources and the delivery of highly personalised services to its 209 million consumers.
2.3 The Importance of Enterprise Modelling in Organizational Strategy
Enterprise Modelling (EM) stands as a fundamental notion in the present business environment, playing a vital role in aligning organizational actions with strategic goals. At its heart, EM gives a holistic picture of an organization's operations, processes, and information systems, operating as a roadmap for strategic alignment (Vernadat 2020). Such a plan assures that all components of a corporation move cohesively towards fulfilling its overarching objectives. A research done by McKinsey in 2022 found that 87% of executives believe that integrating business models with organizational strategies is crucial for attaining competitive advantage, while just 23% think they are effective at doing so (Guggenberger, Maor and Park, 2023). This gap emphasises the underutilization of enterprise modelling's potential, a subject examined by Hofmann and zu Knyphausen?Aufseß (2022). According to them, enterprise modelling can bridge the gap between strategic vision and operational execution by offering clarity on how resources, activities, and skills connect. Furthermore, Lange et al. (2023) underline EM as a crucial instrument for organizational agility. In continually changing markets, having a robust yet flexible corporate architecture allows organisations to pivot their strategy successfully while preserving alignment with their key objectives.
Netflix, as an industry pioneer, highlights the immense importance of great business modelling. With a dynamic content collection, a huge array of user preferences, and extensive technological infrastructure, the streaming giant necessitates a complete EM framework. This guarantees that numerous divisions, from content acquisition to tech development, correspond with the overall strategy objectives. In the context of Netflix, enterprise modelling has permitted its worldwide expansion, enabling it to appeal to various audiences while retaining a consistent corporate brand and user experience. The implementation of EM into Netflix's corporate structure has definitely been a cornerstone in its continued international success.
2.4 DRM Best Practices in the Entertainment Industry
In the entertainment business, the successful use of Data Resource Management (DRM) best practices has become vital. With the introduction of digital platforms and the expanding volume of data they produce, there's a greater demand to handle this data safely and profitably. One of the cornerstones of DRM best practices, as stressed by Stevens (2019), is the principle of data integrity and accuracy. With the entertainment business depending largely on user analytics for decision-making, keeping data accuracy is non-negotiable. Moreover, the GDPR compliance and other data protection requirements further underscore the demand for data integrity and security. An insightful research by PwC in 2022 indicated that 65% of entertainment sector leaders regarded DRM as a critical challenge in their business strategy (PwC, 2020). This awareness of DRM's significance has fostered a number of best practices, such as regular data audits, effective encryption systems, and broad data governance frameworks. Bühler et al. (2023) elaborates on this, underlining the need of a good governance structure that delineates roles, responsibilities, and access rights, so insuring that data is both accessible and safeguarded.
Additionally, Rigby, Sutherland and Takeuchi (2022) say that another outstanding technique rests in the agile management of data. With the fast-paced evolution of the entertainment sector, organisations want DRM methods that can react rapidly to changing business needs and future technologies. For Netflix, the manifestation of these best practices is evident. With a vast worldwide audience and an ever-evolving material inventory, good DRM approaches are not merely advantageous but critical. By maintaining data integrity, Netflix assures that its recommendation systems stay trustworthy. By establishing a solid governance system, it insures that user data is safeguarded while nevertheless being used to enhance the watching experience. Furthermore, Netflix's adaptive approach to DRM, which corresponds with Larson's emphasis on agility, has enabled it to stay ahead of the curve, reinforcing its position as a worldwide entertainment leader.
3.0 Results
3.1 Efficacy of Data Modelling
Data modelling, a core component of Data Resource Management, plays a significant role in the running and competitiveness of streaming platforms. Netflix, as an industry leader, provides a fascinating case study in its implementation and efficacy. Firstly, data modelling is vital to Netflix's content recommendation engine. According to Fleischman et al. (2021), Netflix's sophisticated algorithms, built upon robust data models, create roughly 75% of all viewer activity. This figure highlights the efficacy of data modelling in personalising user experience. The advanced models detect trends from enormous databases, offering highly personalised content suggestions, which, in turn, enhance viewer engagement and retention. van Es (2020) illustrates that the intricacy of Netflix’s data models extends beyond fundamental watching patterns. They account for features such viewing times, search behavior, and even pause or rewind activities. Such depth in data modelling permits for nuanced insights into user behavior, which is subsequently leveraged to improve platform functionality and content curation.
Moreover, Netflix's attention to enhancing its data models has contributed to technical breakthroughs in the sector. As reported by Tagliabue et al. (2021), Netflix produced a product named Metaflow in 2019. This application was built to facilitate the process of building and distributing data models, reflecting Netflix's drive to consistently improve its data management infrastructure. In the standpoint of efficacy, the real-world influence of Netflix's data modelling may be observed in its global success and membership retention. The platform’s flexibility to personalise content to various international audiences and its always improving user experience are a credit to the efficiency of its data modelling efforts. In short, Netflix’s approach to data modelling creates a gold standard in the streaming business, resulting into genuine economic gains and better user experience.
3.2 Data-Enterprise Model Integration Challenges
Integrating data models with enterprise models is a demanding endeavour, even for industry frontrunners like Netflix. Such integration projects, while crucial for acquiring a full operational picture, come with their particular set of difficulties. A big challenge mentioned by Statista (2023) is the volume and velocity of data at Netflix. With over 238.39 million consumers globally streaming media continually, the volume of data generated is huge. While this data is crucial, integrating it into enterprise-wide activities demands complicated data models and infrastructures. Managing this ongoing input, and ensuring its smooth incorporation into bigger business strategies, is no trivial effort. Furthermore, according to Steck et al. (2021), the diverse range of data sources at Netflix causes another challenge. Data floods in from multiple touchpoints, including user interfaces, customer feedback, content performance indicators, and third-party connectivity. Streamlining such disparate data into a uniform organisational model involves sophisticated data integration technologies and processes.
Yet second problem derives from the growing character of global marketplaces. As per a research by Nam, Ro and Jung (2023), 60% of worldwide streaming platforms, including Netflix, have had to adapt their models often in response to regional content tastes, regulatory changes, or technical upgrades. Such constant adaptation could strain the data-enterprise model integration, needing regular recalibrations.
Despite these challenges, Netflix's determination to overcome integration problems is clear. Their investment in technology, especially their real-time data processing tool called Flink, indicates their focus to optimising the integration process. However, while the streaming giant has made remarkable successes, the issues of data-enterprise model integration serve as a reminder of the subtleties inherent in handling enormous and different data in a dynamic global context.
3.3 Competitive Advantage through DRM
Netflix's dominance in the streaming context is not simply owing to its vast content collection or branding, but significantly to its purposeful implementation of Data Resource Management (DRM). In-depth analysis finds how DRM has directly helped to Netflix's competitive edge in the industry. A noteworthy component of Netflix's DRM is its user recommendation system. According to a report by Singh (2022), the platform's algorithm-driven content suggestions account for nearly 80% of the information consumed by subscribers. This high proportion indicates the strategic expertise with which Netflix deploys its data models, guaranteeing viewers are continually engaged with tailored content, hence aiding in subscriber retention. Further enhancing Netflix's standing is its adeptness at predictive analytics. As highlighted out by Kasula (2022), Netflix spends heavily on DRM to predict trends, projecting what material genres or subjects will be popular in the future. Such foresight, rooted on complex data models, allows Netflix to make smart judgements on content creation and acquisition, maintaining a step ahead of competitors.
Moreover, DRM plays a function in Netflix's planned global expansion. Turon (2022) highlights how Netflix leverages geographically segmented data to modify content offers to regional needs. Such granularity in data consumption assures that Netflix communicates with distinct international populations, making it a popular across many areas. Netflix's approach to DRM transcends standard data management. Instead, it designs strategies founded in rich data insights, placing the business at the vanguard of the streaming industry. Through DRM, Netflix doesn't simply adapt to the market but frequently sets its patterns, illustrating the potential of strategic DRM in imparting a considerable competitive advantage.
3.4 Scalability Concerns
As streaming firms grow their reach, scalability emerges as a serious concern, with Netflix being no exception. The platform's exponential expansion has brought to the forefront difficulties and concerns vital to scalability. An important admission by Schaffner et al. (2023) suggests that Netflix handles over 1 billion hours of content streamed per week. Such numbers underscore the massive data throughput and infrastructural issues faced by the streaming behemoth. Accommodating this magnitude involves not just vast storage and processing capacity but also good Data Resource Management to protect data quality and timeliness. Further complicating difficulties is the multitude of Netflix's service options. As stated by Scott (2023), Netflix's shift from a content distributor to a content producer has broadened its data streams. The platform now needs to manage data from production timetables, multinational content distribution networks, and even social media buzz surrounding its original shows. This multi-faceted data ecosystem demands scalable DRM solutions that can manage expanding complexity.
Additionally, Netflix's international presence adds layers to scaling challenges. As stated by Lotz (2022), Netflix operates in over 190 countries, each with its distinct content tastes, regulatory frameworks, and user behavior patterns. Adapting DRM techniques to such varied circumstances while retaining a unified international service poses inherent scale challenges. In light of these hurdles, Netflix's approach to scalability stands as a credit to its imaginative spirit. Investments in cutting-edge technologies, notably the distributed database system Apache Cassandra, reflect its attention to scalability (Ahnert et al. 2023). Nonetheless, as Netflix maintains its international domination and variety, scaling remains a dynamic problem, illustrating the difficulty of managing huge data resources in a continually rising digital economy.
4.0 Discussion
Netflix's standing as a leader in the streaming business is unquestioned. Yet, the results show that its success doesn't only hinge on content but largely on good results Resource Management (DRM). The platform’s sophisticated user recommendation algorithms, predictive analytics, and tailored regional content strategies are grounded in DRM. Such substantial reliance underscores the criticality of DRM in defining user experience and directing corporate strategy. However, there exist obstacles. While the efficacy of data modelling at Netflix boosts user engagement, the integration of these data models with bigger organisational strategy exposes issues. Handling massive volumes of data and combining them with overarching company goals is a demanding task. The different data sources further aggravate integration challenges, necessitating a seamless mesh of complex data models with enterprise-wide frameworks. This equilibrium between micro-level data management and macro-level strategy building remains fragile. Netflix's DRM-driven competitive advantage is another topic demanding further scrutiny. While its data-driven activities help it to remain ahead of competition, such an edge can be transitory. As competitors increase their DRM tactics and as technology continually evolves, Netflix's present lead can vanish. Thus, constant innovation and adaptation in DRM are important. Scalability difficulties provide another layer of complexity. Netflix's international spread and various service offerings demand scalable DRM solutions. However, when service increases, assuring continual data veracity, integrity, and responsiveness becomes hard. Addressing these scalability problems while keeping service quality is a tightrope walk. In essence, whereas Netflix highlights the merits of strategic DRM, it also showcases the inherent drawbacks. The mix of comprehensive data management, corporate strategy, and scalability produces a challenging environment for future study and understanding.
Suggestions for Future Work
Future study should delve further into the evolving nature of DRM technologies and their effect on company strategy, especially as rivals adapt and the technological environment advances. There's an untapped potential in exploring how forthcoming technologies, like quantum computing or advanced AI, might affect DRM regulations in streaming services. Furthermore, a comparative analysis between Netflix and developing rivals would give insights into alternative DRM solutions and their efficacies. Analyzing the effect of international legislation changes on DRM, especially with rising concerns regarding data privacy, could be significant. Lastly, given the scaling limitations highlighted, future study may focus on novel solutions that handle the challenges of managing data resources in ever-expanding digital ecosystems. This line of inquiry could give valuable information for platforms striving to develop without compromising on data quality and timeliness.
References
Abid, H., 2022. A review on the most common pricing strategies. International Journal of Finance, Insurance and Risk Management. Available at: https://scholar.archive.org/work/7didhrktjfcivaawwdas5qiese/access/wayback/https://files.osf.io/v1/resources/a274h/providers/osfstorage/6251cc28be9ffc15b1a03147?action=download&direct&version=1 (Accessed: 11 October 2023).
Ahnert, R., Griffin, E., Ridge, M. and Tolfo, G., 2023. Collaborative Historical Research in the Age of Big Data: Lessons from an Interdisciplinary Project. Elements in Historical Theory and Practice. Available at: https://www.cambridge.org/core/elements/collaborative-historical-research-in-the-age-of-big-data/839C422CCAA6C1699DE8D353B3A1960D (Accessed: 11 October 2023).
Bertoni, A., Hallstedt, S.I., Dasari, S.K. and Andersson, P., 2021. Integration of value and sustainability assessment in design space exploration by machine learning: an aerospace application. Design Science, 6, p.e2. Available at: https://www.cambridge.org/core/journals/design-science/article/integration-of-value-and-sustainability-assessment-in-design-space-exploration-by-machine-learning-an-aerospace-application/B1AE5C87B85ECD9D21D2282E182E538B (Accessed: 11 October 2023).
Bibri, S.E., 2022. Compact urbanism and the synergic potential of its integration with data-driven smart urbanism: An extensive interdisciplinary literature review. Land Use Policy, 97, p.104703. Available at: https://www.sciencedirect.com/science/article/abs/pii/S0264837719317429 (Accessed: 11 October 2023).
Bühler, M.M., Calzada, I., Cane, I., Jelinek, T., Kapoor, A., Mannan, M., Mehta, S., Mookerje, V., Nübel, K., Pentland, A. and Scholz, T., 2023. Unlocking the power of digital commons: Data cooperatives as a pathway for data sovereign, innovative and equitable digital communities. Digital, 3(3), pp.146-171.Available at: https://www.mdpi.com/2673-6470/3/3/11 (Accessed: 11 October 2023).
Deloitte (2022) Digital Media Trends Survey, 14th edition, Deloitte Insights. Available at: https://www2.deloitte.com/us/en/insights/industry/technology/digital-media-trends-consumption-habits-survey/summary-2020.html (Accessed: 11 October 2023).
Fleischman, B., Ondracek, J., Saeed, M. and Bertsch, A., 2021. Netflix: Strategizing Corporate Resources and Capabilities. Available at: https://www.indianjournals.com/ijor.aspx?target=ijor:sijp&volume=8&issue=4&article=008 (Accessed: 11 October 2023).
Gartner (2022) 12 data and analytics trends for times of uncertainty, Gartner. Available at: https://www.gartner.com/en/articles/12-data-and-analytics-trends-to-keep-on-your-radar (Accessed: 11 October 2023).
Guggenberger, P., Maor, D. and Park, M. (2023) The state of organizations 2023: Ten shifts transforming organizations, McKinsey & Company. Available at: https://www.mckinsey.com/capabilities/people-and-organizational-performance/our-insights/the-state-of-organizations-2023 (Accessed: 11 October 2023).
Hofmann, F. and zu Knyphausen?Aufseß, D., 2022. Circular business model experimentation capabilities—A case study approach. Business Strategy and the Environment, 31(5), pp.2469-2488. Available at: https://onlinelibrary.wiley.com/doi/full/10.1002/bse.3038 (Accessed: 11 October 2023).
Kasula, C.P. (2022) Netflix Recommender system?-?A big data case study, Medium. Available at: https://towardsdatascience.com/netflix-recommender-system-a-big-data-case-study-19cfa6d56ff5 (Accessed: 11 October 2023).
Lange, F., Tomini, N., Brinkmann, F., Kanbach, D.K. and Kraus, S., 2023. Demystifying massive and rapid business scaling–An explorative study on driving factors in digital start-ups. Technological Forecasting and Social Change, 196, p.122841. Available at: https://www.sciencedirect.com/science/article/pii/S0040162523005267 (Accessed: 11 October 2023).
Lavin, A., Gilligan-Lee, C.M., Visnjic, A., Ganju, S., Newman, D., Ganguly, S., Lange, D., Baydin, A.G., Sharma, A., Gibson, A. and Zheng, S., 2022. Technology readiness levels for machine learning systems. Nature Communications, 13(1), p.6039. Available at: https://www.nature.com/articles/s41467-022-33128-9 (Accessed: 11 October 2023).
Lotz, A.D., 2022. In between the global and the local: Mapping the geographies of Netflix as a multinational service. International Journal of Cultural Studies, 24(2), pp.195-215. Available at: https://journals.sagepub.com/doi/full/10.1177/1367877920953166 (Accessed: 11 October 2023).
Mololoth, V.K., Saguna, S. and Åhlund, C., 2023. Blockchain and machine° learning for future smart grids: A review. Energies, 16(1), p.528. Available at: https://www.mdpi.com/1996-1073/16/1/528 (Accessed: 11 October 2023).
Montoro?Pons, J.D., Caballer?Tarazona, M. and Cuadrado?García, M., 2021. From pirates to subscribers: 20 years of music consumption research. International Journal of Consumer Studies, 45(4), pp.690-718. Available at: https://onlinelibrary.wiley.com/doi/abs/10.1111/ijcs.12660 (Accessed: 11 October 2023).
Nam, J., Ro, D. and Jung, Y., 2023. Netflix's presence: Investigating content producers' understanding of Netflix in the Korean media industry. Telecommunications Policy, 47(4), p.102525. Available at: https://www.sciencedirect.com/science/article/abs/pii/S0308596123000368 (Accessed: 11 October 2023).
PwC (2022) Pulling the future forward: The entertainment and media industry reconfigures amid recovery, PwC. Available at: https://www.pwc.com/gx/en/news-room/press-releases/2020/global-entertainment-media-outlook-2020.html (Accessed: 11 October 2023).
Rigby, D., Sutherland, J. and Takeuchi, H. (2022) Embracing agile, Harvard Business Review. Available at: https://hbr.org/2016/05/embracing-agile (Accessed: 11 October 2023).
Schaffner, B., Stefanescu, A., Campili, O. and Chetty, M., 2023. Don't Let Netflix Drive the Bus: User's Sense of Agency Over Time and Content Choice on Netflix. Proceedings of the ACM on Human-Computer Interaction, 7(CSCW1), pp.1-32. Available at: https://dl.acm.org/doi/abs/10.1145/3579604 (Accessed: 11 October 2023).
Scott, G., 2023. Navigating the Tides of Change: Global Media Trends in the 21st Century. Global Media Journal, 21(64), pp.1-4. Available at: https://www.proquest.com/openview/402f0f5c7d2060be537a7c5ee9ef818f/1?pq-origsite=gscholar&cbl=29638 (Accessed: 11 October 2023).
Singh, A., 2022. Interplay of the Growing Digital Media and Entertainment Industry with Competition Laws. Supremo Amicus, 30, p.502. Available at: https://heinonline.org/HOL/Page?handle=hein.journals/supami30&div=49&g_sent=1&casa_token= (Accessed: 11 October 2023).
Statista (2023) Netflix: Number of subscribers worldwide 2023, Statista. Available at: https://www.statista.com/statistics/250934/quarterly-number-of-netflix-streaming-subscribers-worldwide/#:~:text=How%20many%20paid%20subscribers%20does,compared%20with%20the%20previous%20quarter. (Accessed: 11 October 2023).
Steck, H., Baltrunas, L., Elahi, E., Liang, D., Raimond, Y. and Basilico, J., 2021. Deep learning for recommender systems: A Netflix case study. AI Magazine, 42(3), pp.7-18. Available at: https://ojs.aaai.org/aimagazine/index.php/aimagazine/article/view/18140 (Accessed: 11 October 2023).
Tagliabue, J., Tuulos, V., Greco, C. and Dave, V., 2021. DAG Card is the new Model Card. arXiv preprint arXiv:2110.13601.
Tshontsho, K.M., 2020. A framework to enhance database models. University of Johannesburg (South Africa). Available at: https://www.proquest.com/openview/a1bc62d9a0333b49d68e218e21e82a42/1?pq-origsite=gscholar&cbl=2026366&diss=y (Accessed: 11 October 2023).
Turon, M. (2022) Netflix’s Secret sauce: How data and analytics propelled ... - linkedin, Linkedin. Available at: https://www.linkedin.com/pulse/netflixs-secret-sauce-how-data-analytics-propelled-them-michael-turon (Accessed: 11 October 2023).
van Es, K., 2023. Netflix & Big Data: The Strategic Ambivalence of an Entertainment Company. Television & New Media, 24(6), pp.656-672. Available at: https://journals.sagepub.com/doi/full/10.1177/15274764221125745 (Accessed: 11 October 2023).
Vernadat, F., 2020. Enterprise modelling: Research review and outlook. Computers in Industry, 122, p.103265. Available at: https://www.sciencedirect.com/science/article/abs/pii/S0166361520304991 (Accessed: 11 October 2023).