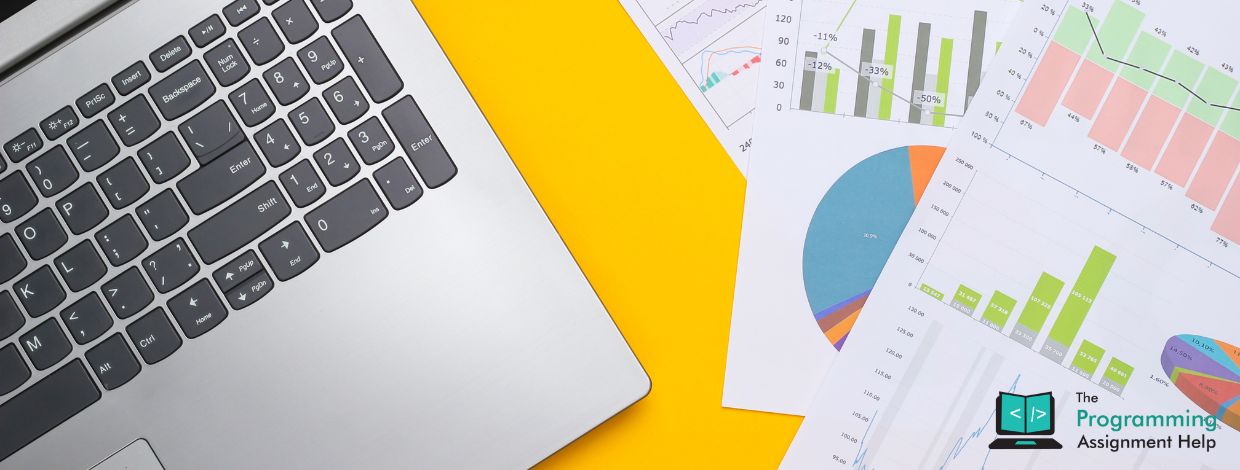
- 18th Jul 2024
- 17:36 pm
- Adan Salman
This assignment aims to give you an idea of applying EDA in a real business scenario. In this assignment, apart from applying the techniques that you have learnt in the EDA module, you will also develop a basic understanding of risk analytics in banking and financial services and understand how data is used to minimise the risk of losing money while lending to customers.
Business Understanding
The loan providing companies find it hard to give loans to the people due to their insufficient or non-existent credit history. Because of that, some consumers use it as their advantage by becoming a defaulter. Suppose you work for a consumer finance company which specialises in lending various types of loans to urban customers. You have to use EDA to analyse the patterns present in the data. This will ensure that the applicants capable of repaying the loan are not rejected.
When a client applies for a loan, there are four types of decisions that could be taken by the client/company):
- Approved: The Company has approved loan Application
- Cancelled: The client cancelled the application sometime during approval. Either the client changed her/his mind about the loan or in some cases due to a higher risk of the client he received worse pricing which he did not want.
- Refused: The company had rejected the loan (because the client does not meet their requirements etc.).
- Unused offer: Loan has been cancelled by the client but on different stages of the process.
In this case study, you will use EDA to understand how consumer attributes and loan attributes influence the tendency of default.
Applying EDA in Risk Analytics for Loan Approval Decisions - Get Assignment Solution
Please note that this is a sample assignment solved by our Python Programmers. These solutions are intended to be used for research and reference purposes only. If you can learn any concepts by going through the reports and code, then our Python Tutors would be very happy.
- To download the complete solution along with Code, Report and screenshots - Please visit our Programming Assignment Sample Solution page
- Reach out to our Python Tutors to get online tutoring related to this assignment and get your doubts cleared
- You can check the partial solution for this assignment in this blog below
Free Assignment Solution - Applying EDA in Risk Analytics for Loan Approval Decisions
__Importing Basic Libraries:__
"""
# Commented out IPython magic to ensure Python compatibility.
import pandas as pd
import numpy as np
import seaborn as sns;sns.set(style="white")
import matplotlib.pyplot as plt
# %matplotlib inline
import warnings
warnings.simplefilter("ignore")
"""__Loading Dataset:__"""
df = pd.read_csv("/content/drive/MyDrive/Colab Notebooks/Classifier/application_data.csv")
df= df.drop("SK_ID_CURR", axis=1)
print("Our orignal data-set have {} rows and {} columns. \n".format(df.shape[0], df.shape[1]))
df.head()
"""__Descriptive Statistics:__"""
df.describe()
"""## Data Visualization:
* __Correlation HeatMap:__
"""
# # I just checked correlated feature with greater than .8 here
# corr = df.corr()
# corr_greater_than_80 = corr[corr>=.8]
# corr_greater_than_80
"""* __Normal Distribution:__"""
df_numerics_only = df.select_dtypes(include=np.number)
cols = df_numerics_only.columns.tolist()
cols.remove("TARGET")
for col in cols:
fig, ax = plt.subplots()
fig.set_size_inches(15, 5)
sns.distplot(df[col], color="m")
"""* __Count Plot:__"""
df_category_only = df.select_dtypes(exclude=np.number)
cols = df_category_only.columns
for col in cols:
fig, ax = plt.subplots()
fig.set_size_inches(15, 5)
sns.countplot(df[col], palette="Set3", hue=df["TARGET"])
plt.xticks(rotation=90)
#Countplot of TARGET variable
f,ax=plt.subplots(1,2,figsize=(18,8)
# Labeling categorical variables.
from sklearn import preprocessing
#label Encoder
df_category_only = df.select_dtypes(exclude=np.number)
category_col = df_category_only.columns
df[category_col] = df[category_col].astype('|S80')
labelEncoder = preprocessing.LabelEncoder()
# creating a map of all the numerical values of each categorical labels.
mapping_dict={}
for col in category_col:
df[col] = labelEncoder.fit_transform(df[col])
le_name_mapping = dict(zip(labelEncoder.classes_, labelEncoder.transform(labelEncoder.classes_)))
mapping_dict[col]=le_name_mapping
print(mapping_dict)
"""* __Preparing X and y using pandas:__"""
X= df.drop("TARGET", axis=1)
y = df["TARGET"]
X_col = X.columns
"""* __Splitting Data into train and test sample:__"""
# Splitting data into train and test sample using 70% data for training and 30% data for testing
from sklearn.model_selection import train_test_split
X_train, X_test, y_train, y_test = train_test_split(X, y, test_size=0.30, stratify=y)
"""* __Treatimg Imbalance in Training Dataset:__"""
from imblearn.over_sampling import SMOTE
sm = SMOTE(random_state = 42)
X_train, y_train = sm.fit_resample(X_train, y_train)
# count of training and validation class
plt.figure(1 , figsize = (25 ,5))
n = 0
for z , j in zip([y_train , y_test] , ['train data', 'test data']):
n += 1
plt.subplot(1 , 3 , n)
sns.countplot(x = z, palette="Set3" )
plt.title(j)
plt.show()
plt.show()
"""* __Naive Bayes:__"""
from sklearn.naive_bayes import GaussianNB
steps = [ ('standard', StandardScaler()) ,('pca', PCA(n_components=10)), ('clf', GaussianNB())]
clf3 = Pipeline(steps=steps)
clf3.fit(X_train, y_train)
predictions= clf3.predict(X_test)
train_acc3 = clf3.score(X_train, y_train)*100
test_acc3 = clf3.score(X_test, y_test)*100
print("Accuracy on training set: {:.3f}%. \n".format(train_acc3))
print("Accuracy on test set: {:.3f}%. \n".format(test_acc3))
print("Classification Report: \n",classification_report(y_test, predictions))
print()
cm = confusion_matrix(y_test, predictions)
print("Confusion Matrix: " )
confusion = pd.DataFrame(cm, columns = map, index = map)
confusion
# calculate scores
ns_probs = [0 for _ in range(len(y_test))]
ns_auc = roc_auc_score(y_test, ns_probs)
lr_auc = roc_auc_score(y_test, predictions)
# summarize scores
print('No Skill: ROC AUC=%.3f' % (ns_auc))
print('Naive Bayes: ROC AUC=%.3f' % (lr_auc))
# calculate roc curves
ns_fpr, ns_tpr, _ = roc_curve(y_test, ns_probs)
lr_fpr, lr_tpr, _ = roc_curve(y_test, predictions)
# plot the roc curve for the model
plt.figure(figsize=(15,6))
plt.plot(ns_fpr, ns_tpr, linestyle='--', label='No Skill')
plt.plot(lr_fpr, lr_tpr, marker='.', label='Naive Bayes')
# axis labels
plt.xlabel('False Positive Rate')
plt.ylabel('True Positive Rate')
# show the legend
plt.legend()
# show the plot
plt.show()
"""* __KNN:__"""
from sklearn.neighbors import KNeighborsClassifier
error_rate = []
# Will take some time
for i in range(1,11):
#Decision Tree
from sklearn.tree import DecisionTreeClassifier
steps = [ ('standard', StandardScaler()) ,('pca', PCA(n_components=10)), ('clf', DecisionTreeClassifier())]
clf5 = Pipeline(steps=steps)
clf5.fit(X_train, y_train)
predictions= clf5.predict(X_test)
train_acc5 = clf5.score(X_train, y_train)*100
test_acc5 = clf5.score(X_test, y_test)*100
print()
print("Accuracy on training set: {:.3f}%. \n".format(train_acc5))
print("Accuracy on test set: {:.3f}%. \n".format(test_acc5))
print("Classification Report: \n",classification_report(y_test, predictions))
print()
cm = confusion_matrix(y_test, predictions)
print("Confusion Matrix: " )
confusion = pd.DataFrame(cm, columns = map, index = map)
confusion
# calculate scores
ns_probs = [0 for _ in range(len(y_test))]
ns_auc = roc_auc_score(y_test, ns_probs)
lr_auc = roc_auc_score(y_test, predictions)
# summarize scores
print('No Skill: ROC AUC=%.3f' % (ns_auc))
print('Desicion Tree: ROC AUC=%.3f' % (lr_auc))
# calculate roc curves
ns_fpr, ns_tpr, _ = roc_curve(y_test, ns_probs)
lr_fpr, lr_tpr, _ = roc_curve(y_test, predictions)
# plot the roc curve for the model
plt.figure(figsize=(15,6))
plt.plot(ns_fpr, ns_tpr, linestyle='--', label='No Skill')
plt.plot(lr_fpr, lr_tpr, marker='.', label='Desicion Tree')
# axis labels
plt.xlabel('False Positive Rate')
plt.ylabel('True Positive Rate')
# show the legend
plt.legend()
# show the plot
plt.show()
"""* __Random Forest__"""
from sklearn.ensemble import RandomForestClassifier
steps = [ ('standard', StandardScaler()) ,('pca', PCA(n_components=10)), ('clf', RandomForestClassifier())]
clf6 = Pipeline(steps=steps)
clf6.fit(X_train, y_train)
predictions= clf6.predict(X_test)
train_acc6 = clf6.score(X_train, y_train)*100
test_acc6 = clf6.score(X_test, y_test)*100
print("Accuracy on training set: {:.3f}%. \n".format(train_acc6))
print("Accuracy on test set: {:.3f}%. \n".format(test_acc6))
print("Classification Report: \n",classification_report(y_test, predictions))
print()
cm = confusion_matrix(y_test, predictions)
print("Confusion Matrix: " )
confusion = pd.DataFrame(cm, columns = map, index = map)
confusion
# calculate scores
ns_probs = [0 for _ in range(len(y_test))]
ns_auc = roc_auc_score(y_test, ns_probs)
lr_auc = roc_auc_score(y_test, predictions)
# summarize scores
print('No Skill: ROC AUC=%.3f' % (ns_auc))
print('Random Forest: ROC AUC=%.3f' % (lr_auc))
# calculate roc curves
ns_fpr, ns_tpr, _ = roc_curve(y_test, ns_probs)
lr_fpr, lr_tpr, _ = roc_curve(y_test, predictions)
# plot the roc curve for the model
plt.figure(figsize=(15,6))
plt.plot(ns_fpr, ns_tpr, linestyle='--', label='No Skill')
plt.plot(lr_fpr, lr_tpr, marker='.', label='Random Forest')
# axis labels
plt.xlabel('False Positive Rate')
plt.ylabel('True Positive Rate')
# show the legend
plt.legend()
# show the plot
plt.show()
"""* __XGB Classifier:__"""
from xgboost import XGBClassifier
steps = [ ('standard', StandardScaler()) ,('pca', PCA(n_components=10)), ('clf', XGBClassifier(gamma=0))]
clf7 = Pipeline(steps=steps)
clf7.fit(X_train, y_train)
predictions= clf7.predict(X_test)
train_acc7 = clf7.score(X_train, y_train)*100
test_acc7 = clf7.score(X_test, y_test)*100
print("Accuracy on training set: {:.3f}%. \n".format(train_acc7))
print("Accuracy on test set: {:.3f}%. \n".format(test_acc7))
print("Classification Report: \n",classification_report(y_test, predictions))
print()
cm = confusion_matrix(y_test, predictions)
print("Confusion Matrix: " )
confusion = pd.DataFrame(cm, columns = map, index = map)
confusion
# calculate scores
ns_probs = [0 for _ in range(len(y_test))]
ns_auc = roc_auc_score(y_test, ns_probs)
lr_auc = roc_auc_score(y_test, predictions)
# summarize scores
print('No Skill: ROC AUC=%.3f' % (ns_auc))
print('Random Forest: ROC AUC=%.3f' % (lr_auc))
# calculate roc curves
ns_fpr, ns_tpr, _ = roc_curve(y_test, ns_probs)
lr_fpr, lr_tpr, _ = roc_curve(y_test, predictions)
# plot the roc curve for the model
plt.figure(figsize=(15,6))
plt.plot(ns_fpr, ns_tpr, linestyle='--', label='No Skill')
plt.plot(lr_fpr, lr_tpr, marker='.', label='Random Forest')
# axis labels
plt.xlabel('False Positive Rate')
plt.ylabel('True Positive Rate')
# show the legend
plt.legend()
# show the plot
plt.show()
"""## Algorithm Comparision"""
comp = {"Algorithm": ["Logistic Regression", "Naive Bayes", "KNN", "Decision Tree", "Random Forest", "XG Boost"],
"Test Score":[test_acc1, test_acc3, test_acc4, test_acc5, test_acc6, test_acc7],
"Train Score":[train_acc1, train_acc3, train_acc4, train_acc5, train_acc6, train_acc7],
"Model":[clf1,clf3, clf4, clf5, clf6, clf7]}
comparision = pd.DataFrame(comp)
comparision["Lag Score"] = abs(comparision["Train Score"] - comparision["Test Score"])
comparision
"""## Prediction Based on the Best Performaing Algorithm
* __Loading Unknown Dataset__
"""
dff = pd.read_csv("/content/drive/MyDrive/Colab Notebooks/Classifier/application_data.csv")
Xx = dff[X_col]
print("Our orignal unknown data-set have {} rows and {} columns. \n" .format(Xx.shape[0], Xx.shape[1]))
Xx.head()
"""* __Treating Nan values for Unknown Dataset__"""
Xx = Xx.fillna(0)
"""* __One Hot Encoding for Unknown Dataset:__ """
for col in Xx.columns:
if col in mapping_dict.keys():
sample = {x.decode('utf-8') : y for x,y in mapping_dict[col].items()}
Xx = Xx.replace({col: sample})
Xx.head()
"""* __Prediction on Unknown Dataset__"""
print("Best Performing Algorithm:",comparision.loc[comparision["Test Score"].idxmax(), 'Algorithm'])
model = comparision.loc[comparision["Test Score"].idxmax(), 'Model']
predictions= np.round(model.predict(Xx))
predictions = pd.DataFrame(predictions, columns= ["Prediction"])
row = pd.DataFrame(dff["SK_ID_CURR"], columns= ["SK_ID_CURR"])
merge = [row, predictions]
pred = pd.concat(merge, axis=1)
print("Our orignal prediction data-set have {} rows and {} columns. \n" .format(pred.shape[0], pred.shape[1]))
pred.to_csv(r'Prediction_Unknown.csv', index=False)
pred.head()
Get the best Applying EDA in Risk Analytics for Loan Approval Decisions Assignment help and tutoring services from our experts now!
About The Author - Rehana Mat
Rehana Mat is an expert in data analytics and financial risk management. With a deep understanding of exploratory data analysis (EDA) and its application in banking and financial services, Rehana excels in identifying patterns and insights from large datasets. Her expertise helps companies make informed decisions, minimize risks, and improve loan approval processes.